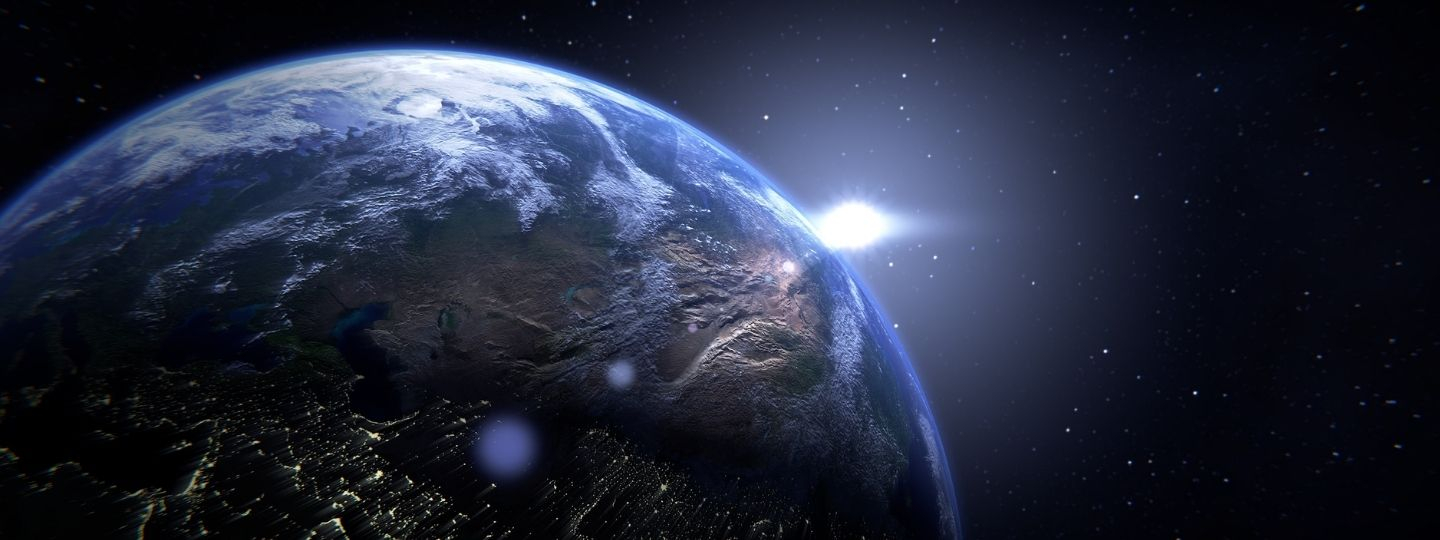
Open Science
Na VUT stojíme za konceptem otevřené vědy. Chceme, aby její výstupy byly jednoduše dostupné, transparentní a snadno ověřitelné.
-
1 189
publikací v databázi Web of Science (za rok 2023)
-
41,63 %
v Q1 časopisech (InCites – dle JIF, 2023)
-
53,32 %
publikací s korespondenčním autorem z VUT (2023)
-
59,49 %
Open Access publikací (2023)
Pressure loss and droplet entrainment under spray absorber conditions
publikaceRok: 2025
CEJPEK, O.; MALÝ, M.; PRINZ, F.; HÁJEK, O.; BĚLKA, M.; LINDOVSKÝ, J.; HÁJEK, J.; NOVOSAD, P.; JEDELSKÝ, J. Pressure loss and droplet entrainment under spray absorber conditions. SEPARATION AND PURIFICATION TECHNOLOGY, 2025, vol. 354, no. 8, ISSN: 1383-5866.
Measurement of electrical conductivity of human tissue: A feasibility study of a novel time-domain approach
publikaceRok: 2025
DOLEŽAL, T.; ŠTUMPF, M.; QIRUI, H.; FRANEK, O. Measurement of electrical conductivity of human tissue: A feasibility study of a novel time-domain approach. MEASUREMENT, 2025, vol. 242, no. Part A,
p. 1-7. ISSN: 1873-412X.Chudoba - Průvodce výstavou
publikaceRok: 2025
ILIČ, B.; KONEČNÝ, M.; POLÁCH, J.; KURTINOVÁ, B.; BODLÁK, O.; LUŇÁK, F.; VOLKOVÁ, V.; BILER, S.; BABÁKOVÁ, B.; RYGÁLOVÁ, M.; DROZD SMUTNÁ, M.; BUTULA CICHÁ, M.; JINDROVÁ, A.; HRADILOVÁ, A. Chudoba - Průvodce výstavou. Chudoba - Průvodce výstavou. Nové Město na Moravě: Horácká galerie v Novém Městě na Moravě, 2025. ISBN: 978-80-87718-20-9.
Druhohorní vulkanismus na Měsíci?
publikaceRok: 2025
KRMÍČEK, L. Druhohorní vulkanismus na Měsíci?. Vesmír, 2025, roč. 104, č. 1,
s. 12-13. ISSN: 0042-4544.Mobilní omezovač rychlosti pro komunikaci mezi vozidly V2V
produktRok: 2025
LOKAJ, Z.; ŠROTÝŘ, M.; VANIŠ, M.; MIKLÓŠIK, I.; MARTINÁSEK, Z.; DVOŘÁK, J.; ŠEDA, P.; POSPÍŠIL, J.; MALINA, L.: VJ01010066-V2; Mobilní omezovač rychlosti pro komunikaci mezi vozidly V2V. Ústav aplikované informatiky v dopravě, FD, ČVUT v Praze a Fakulta elektrotechniky a komunikačních technologií, VUT v Brně. (funkční vzorek)
Singular non-autonomous (p,q)-equations with competing nonlinearities
publikaceRok: 2025
PAPAGEORGIOU, N.; QIN, D.; RADULESCU, V. Singular non-autonomous (p,q)-equations with competing nonlinearities. NONLINEAR ANALYSIS-REAL WORLD APPLICATIONS, 2025, vol. 81, no. 104225, ISSN: 1878-5719.
České spotřební pohádky
publikaceRok: 2025
RYŠKA, P. České spotřební pohádky. Brno: Vysoké učení technické v Brně, Fakulta výtvarných umění, 2025. 272 s. ISBN: 978-80-214-6269-4.
Foreseeing wearing-off state in Parkinson’s disease patients, a multimodal approach with the usage of machine learning and wearables
publikaceRok: 2025
SKIBIŃSKA, J.; SHAH, A.; CHANNA, A.; SHAH SYED, M.; SYED, Z.; HOŠEK, J. Foreseeing wearing-off state in Parkinson’s disease patients, a multimodal approach with the usage of machine learning and wearables. In Activity, Behavior, and Healthcare Computing. 1. Taylor and Francis, 2025.
p. 1-14. ISBN: 9781032648422.Vyhodnocovací modul pro dynamické měření jasu do stávajícího softwarového řešení LumiDISP
produktRok: 2025
ŠKODA, J.: DyLDA; Vyhodnocovací modul pro dynamické měření jasu do stávajícího softwarového řešení LumiDISP. Vysoké učení technické v Brně/ UEEN. URL: https://www.lumidisp.eu. (software)
Antibiotická rezistence a struktura populace Flavobacterium psychrophilum v chovech lososovitých ryb v České republice
publikaceRok: 2025
VAIBAROVA, V.; KRÁLOVÁ, S.; PALÍKOVÁ, M.; SCHWARZEROVÁ, J.; ČEJKOVÁ, D.; ČÍŽEK, A. Antibiotická rezistence a struktura populace Flavobacterium psychrophilum v chovech lososovitých ryb v České republice. Veterinářství. Profi Press, s.r.o. GJ, 2025, roč. 75, č. 1,
s. 36-41. ISSN: 0506-8231.Unveiling HDAC8 antagonists for breast cancer therapy via molecular modeling
publikaceRok: 2025
Vaishali Pankaj, Inderjeet Bhogal, Sudeep Roy. Unveiling HDAC8 antagonists for breast cancer therapy via molecular modeling. In 2024 IEEE International Conference on Bioinformatics and Biomedicine (BIBM). IEEE-International Conference on Bioinformatics and Biomedicine (BIBM). Lisbon, Portugal: IEEE, 2025.
p. 852-855. ISBN: 979-8-3503-8622-6. ISSN: 2156-1133.Oponentský posudek projektu KEGA 009TUKE-4/2022
publikaceRok: 2025
VENKRBEC, V. Oponentský posudek projektu KEGA 009TUKE-4/2022. Brno: 2025.
s. 1-2. English for Writing a Bachelor's Thesis: A Guide for BUT Students
publikaceRok: 2025
VRÁNOVÁ, M. English for Writing a Bachelor's Thesis: A Guide for BUT Students. English for Writing a Bachelor's Thesis: A Guide for BUT Students. 1. Brno: Vysoké učení technické v Brně, 2025.
p. 1-109. ISBN: 978-80-214-6307-3.A Tale of Two Desktops: The First Czech Films in Parallel Worlds
publikaceRok: 2025
ŽÁK, J.; HANÁKOVÁ, V.; ANGER, J. A Tale of Two Desktops: The First Czech Films in Parallel Worlds. MOVIE: A Journal of Film Criticism, 2025, no. 12, ISSN: 2047-1661.
Recenze na článek AKTUÁLNÍ STAV SÍTĚ CZEPOS A DATABÁZE BODOVÝCH POLÍ
publikaceRok: 2025
Recenze na článek AKTUÁLNÍ STAV SÍTĚ CZEPOS A DATABÁZE BODOVÝCH POLÍ. 2025.
Zobrazeny výsledky 1 - 15 z (ze) 10000 výsledků.
Odpovědnost: Ing. Zdeňka Pavlačková, Ph.D.